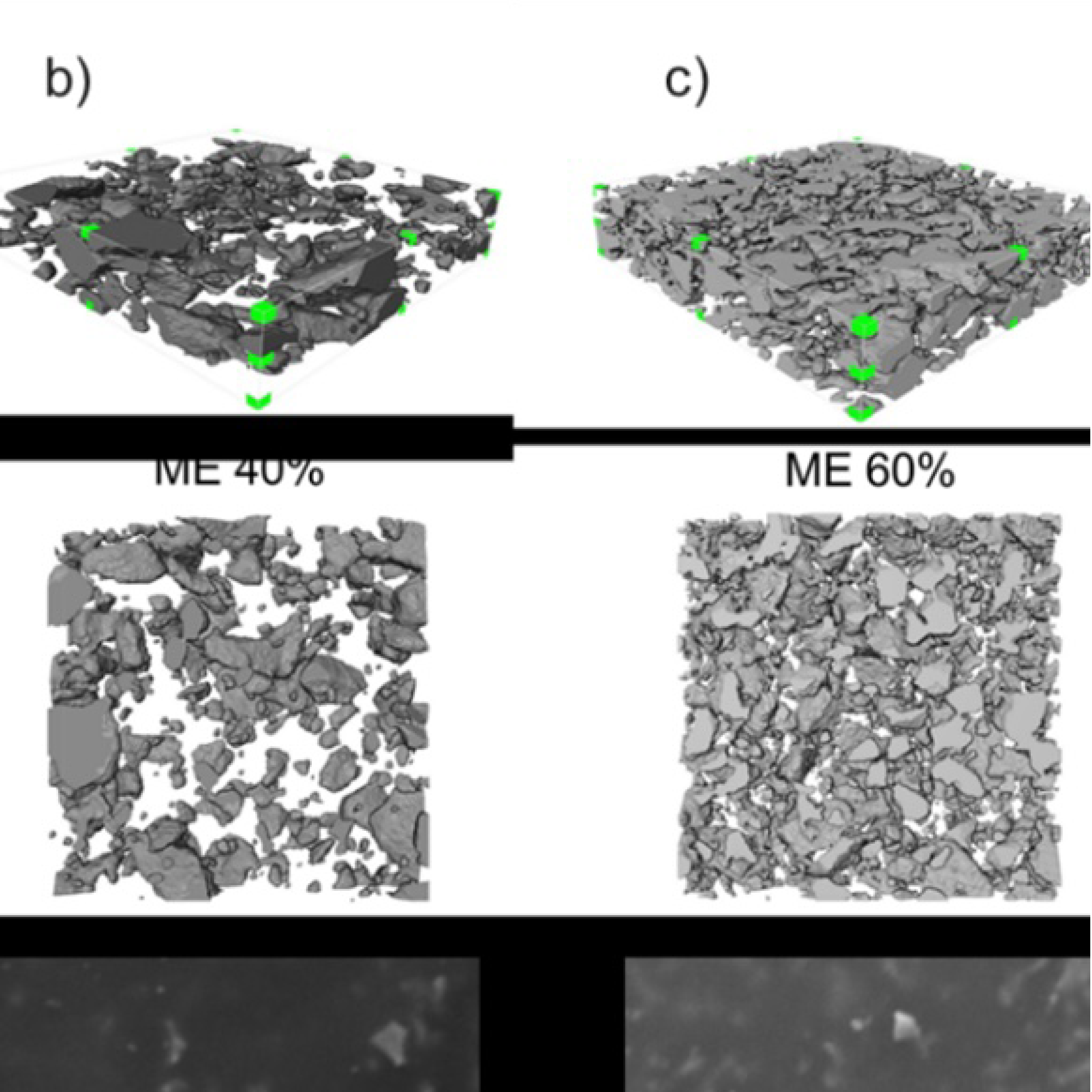
3D Microstructure of Soft Magnetic Elastomer Membrane
Soft magnetic elastomer membranes enable fast magnetic actuation under low fields. In our project, we… Read More
Events & Resources
News, Events and Resources from NXCT Partners
Background
Accurate permeability data is key to high-fidelity resin flow simulations for composites manufacturing with liquid moulding, yet remains an unsolved challenge due to variabilities at different length scales, for which comprehensive 3D characterisations are required. X-CT is an ideal tool for this purpose and is becoming viable for industrial applications. However, the high-resolution scans, needed for accurate permeability calculations, are usually time consuming and generate huge datasets when covering large enough fields of view. This work will develop deep learning (DL) models that use low-resolution X-CT images to accurately predict the permeability of textile fabrics. This DL model will loosen the requirement of high-resolution scans for permeability assessment. Consequently, it will significantly accelerate the inspection time and decrease the size of necessary X-CT data.
Role of µCT
To generate the training data for these DL models, it is crucial that images of the same region of interest at different resolutions (1~30um/voxel) are obtained. The highest resolution X-CT images will be input into a high-fidelity simulator to predict permeability. DL models will then be trained based on the images of different resolutions and the calculated permeability. The development of the DL model is still ongoing work. The dataset produced at µ-VIS X-Ray Imaging Centre has been helpful for testing our image-based simulation for permeability calculation.
Future impact
The methodology proposed in this work can be readily adopted by other scientific and engineering endeavours where the problem of porous-medium flow is pivotal, e.g. fuel cells, carbon storage, nuclear waste disposal, etc. For the latter areas, the present work will stimulate other exciting research, and serve as a proof-of-concept study supporting future grant applications.
“The deep learning (DL) models once trained in this work will has the potential to become a standard universal tool for composite manufacturing. This DL model will loosen the requirement of high-resolution scans for permeability assessment. Consequently, it will significantly accelerate the inspection time and decrease the size of necessary X-CT data. This work will be an important proof-of-concept study for many new applications of DL in engineering problems.”
Yang Chen
Research Fellow
Department of Mechanical Engineering, University of Bath
Figure 1. Three images with different resolutions of the same composite samples: top – cross-sectional slice, bottom – 3D view of the NCF sample.
Figure 2. Image-based simulation for the permeability prediction of the NCF sample: left – segmented image, where the fibre tows with different orientations coloured differently; middle – local fibre orientations determined with a structure-tensor based approach; right – velocity field calculated using an FFT solver for Stokes-Brinkman equation.
Soft magnetic elastomer membranes enable fast magnetic actuation under low fields. In our project, we… Read More
Nowadays, the increasing capability of micro-manufacturing processes enables the manufacture of miniature products with extremely… Read More
Injection of CO2 into shale reservoirs to enhance gas recovery and simultaneously sequester greenhouse… Read More